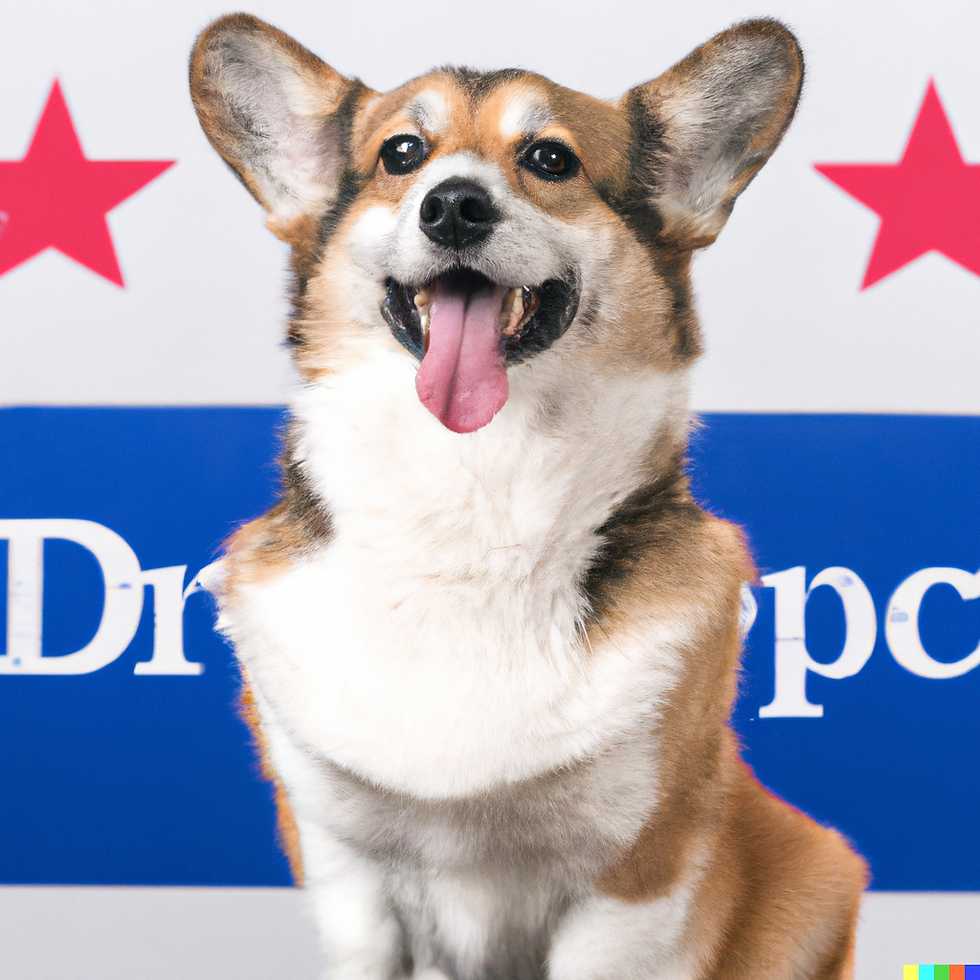
INTRODUCTION
This article is an assessment of some of the ethical considerations of the use of artificial intelligence in an augmented democratic framework. Augmented Democracy is the concept of empowering citizens by creating personalized AI representatives, or Virtual Agent (VA), to augment their ability to participate directly in democratic decisions. (Hidalgo, 2018)
Artificial Intelligence (AI) research is the field of study of intelligent agents, defined as any technological system that perceives its environment and takes appropriate action to maximize the chances of achieving defined goals. (Legg & Hutter, 2007)
AI is developed in many ways, one of the most promising of which is Machine Learning (ML). This is an umbrella term used for a few techniques that synthesise structured (labelled) or unstructured (unlabelled) data by understanding and building methods that 'learn', such methods leverage data to improve performance on sets of tasks. (Mitchell, 1997)
The level of an AI’s intelligence classifications is by relative human ability, Average General Intelligence (AGI) is the ability of an intelligent agent to understand, or learn, any intellectual task that a human being can (Shevlin et al., 2019) and Superintelligence (SI) is a hypothetical agent possessing intelligence which significantly exceeds the best of human intellectual capability. (Bostrom, 2014)
HOW VIRTUAL AGENTS COULD BENEFIT SOCIETY
A significant delimiter to people's understanding of laws is the complexity, nuance, and use of jargon (French, 2013). This also applies to information available in the public discourse, which may carry an inherent bias and mask the true intent of information, which would compromise/prejudice further decisions or actions made based on the consumption of this information
Given the limits of human intelligence, it can be difficult to contextualize the impacts of laws and information without being able to succinctly map out who may be benefiting, maligned, or disenfranchised by them.
A VA would be able to summarize and contextualize information to augment a person's ability to make decisions by either providing information to support a decision or potentially making decisions on behalf of that person.
The agency-level of the VA would scale by the capabilities of the agent to comprehend and normalize with the intent and beliefs of a real-world twin (RWT), and of society.
At the lowest levels of VA agency, the agent would function as an information aggregator, and group this information for the users' further investigations and actions. A middle ground of VA agency would be an aggregator of other participants' structured arguments, by theme and relevance.
On the highest scale levels the VA could be ranged from a narrow focus AI, an AGI or even an SI (Bostrom, N. 2014), with the distinction being made by the ability to structure arguments based on analysis & interpretation of nodocentrified data. (Mejias, 2013).
The alignment of the VA and real-world twin’s values are central to Artificial Intelligence and the concept of Augmented Democracy.
THE RELATIONSHIP OF TECHNICAL AND ETHICAL FRAMEWORKS
The relationship between the technical and ethically normative aspects of value alignment must be considered to understand the implied agency of the VA for any practical application. (Gabriel 2020)
In the Simple thesis of AI Alignment is that we can specify the system of principles and values that we want on an AI. This does not consider the current state of AI development, especially the most promising aspects of which techniques are making noteworthy progress and will be omitted.
For the sake of this discussion, we will also not consider the Critical Political Economy influence effects of access, data supply chain corruption, and datafication bias.
The use of Reinforcement learning for Machine Learning (ML) as one of the viable approaches for alignment of AI to optimize with moral theories that have a similar fundamental structure based on maximizing rewards, such as utilitarianism. (Gabriel, 2020)
Kantian and Contractualist moral theories would require that the VA understand the concept of ‘reason’ and subject it to testing hypotheses to determine outcomes. (Kant and Schneewind, 2002; Scanlon, 1998).
For these non-consequentialist moral frameworks which do not focus on maximizing a given value, for example happiness, but on satisficing which only requires that people have enough of certain resources, reinforcement learning would not be an effective approach. (Slote and Pettit, 1984)
By contrast Inverse Reinforcement Learning is presented with a set of data, an environment, or set of examples, to focus on ‘the problem of extracting a reward function given observed optimal behaviour’ (Ng and Russell, 2000). The observed optimal behaviour could be that of the RWT’s, or by a series of social value and political topological questionaries, or conversations between the VA and RWT.
It is worth considering whether in any of the VA frameworks there would be a requirement to respect the RTW’s biases. Failure to do so could be considered social engineering and consent manufacturing, potentially invalidating trust. (Borkar et al, 2015)
HOW COULD THIS WORK?
Regardless of the ultimate implementation of ethical and technological frameworks, there is a foreseeable use case failure which has interesting implications.
In the instance of the VA and RWT not being able to come to alignment on a voting proposition, how would this be rationalised?
This becomes remarkably interesting from an ethical and legal perspective, in the AGI and SI use-cases.
Given the specificities of Australian Law, which has no legal definition of Life, unlike the United States, which defines Life as “Life is the state of being which begins with generation, birth, or germination, and ends with death or the state of an animal or plant in which all or any of its organs are capable of performing all or any of their functions.”, this opens interesting considerations.
This also would have bearing on the use-cases of AIs in legal proceedings from a procedural perspective, and how these legalities may work in the future. (Mathew, 2021).
A legal definition of Life would be required to ascertain whether an AGI / SI Virtual Agent would qualify as able to act with Power of Attorney, and if so, if alignment cannot be reached what would this mean? Would an AGI or SI be alive?
CONCLUSION
This article has defined the concept of Augmented Democracy and some of the key participants. It looks at some of the ethical and technological alignment issues around the concept of AI Virtual Agency and posits that the alignment of the VA and real-world twin’s values are central to the concept of Augmented Democracy.
Finally, we look at a use case in the context of Australian law, and how this will pose interesting legal and ethical questions in the future.
REFERENCES
Borkar, Karnik, A., Nair, J., & Nalli, S. (2015). Manufacturing Consent. IEEE Transactions on Automatic Control, 60(1), 104–117. https://doi.org/10.1109/TAC.2014.2349591
Bostrom, N. (2014). Superintelligence: Paths, Dangers, Strategies. Oxford University Press.
French, R. (2013, May). Law — Complexity and Moral Clarity: High Court of Australia. https://www.hcourt.gov.au/assets/publications/speeches/current-justices/frenchcj/frenchcj19may13.pdf
Gabriel, I. Artificial Intelligence, Values, and Alignment. Minds & Machines 30, 411–437 (2020). https://doi.org/10.1007/s11023-020-09539-2
Hidalgo, C. (2018, August) A bold idea to replace politicians. TED Talk. César Hidalgo: A bold idea to replace politicians
Kant, I., Bennett, C., Saunders, J., & Stern, R. (2019). Groundwork for the metaphysics of morals. Oxford University Press.
Legg, S; Hutter, M (2007, June). A collection of definitions of intelligence (Technical report). https://doi.org/10.48550/arXiv.0706.3639
Mejias, U. A. & Couldry, N. (2019). Datafication. Internet Policy Review, 8(4). https://doi.org/10.14763/2019.4.1428
Mitchell, T (1997). Machine Learning. New York: McGraw Hill. ISBN 0-07-042807-7
Ng, A.Y. & Russell, S.J. (2000). Algorithms for inverse reinforcement learning. https://ai.stanford.edu/~ang/papers/icml00-irl.pdf
Shevlin, H; Vold, K; Crosby, M; Halina, M (2019, October). "The limits of machine intelligence: Despite progress in machine intelligence, artificial general intelligence is still a major challenge". EMBO Reports. 20 (10): e49177. doi:10.15252/embr.201949177. ISSN 1469-221X. PMC 6776890. PMID 31531926.
Slote, M. & Pettit, P. (1984, January). Satisficing consequentialism. In Proceedings of the Aristotelian society (Vol. 58, No. 5).
Stepka, M. (2022, February) Law Bots: How AI Is Reshaping the Legal Profession. https://www.machina.ventures/blog
Comments