Part 1.
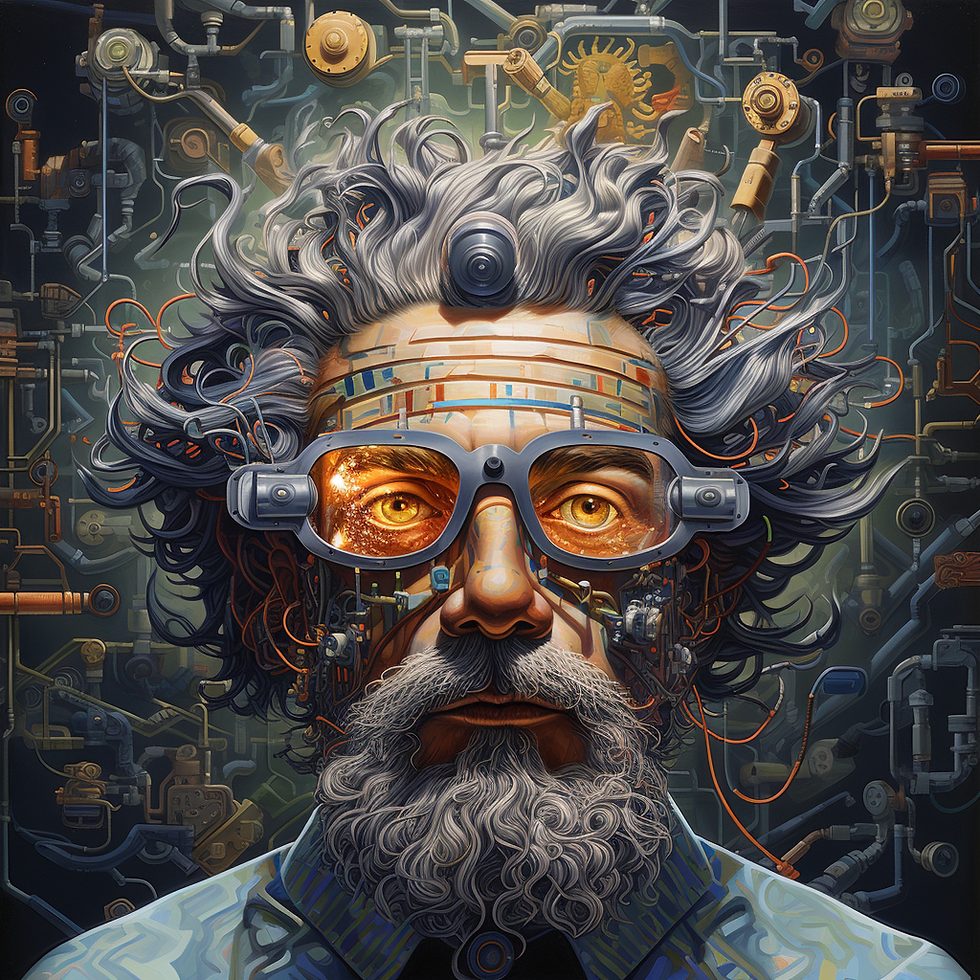
Decision intelligence (DI) is a multidisciplinary field that encompasses data science, behavioral science, social science, and managerial science to improve decision-making.
It's a framework that uses a variety of techniques and technologies, such as machine learning, AI, and big data analytics, to help decision-makers choose the most effective actions given their conditionalities and goals.
The term, and field, come from the work by Cassie Kozyrkovs, Google's chief decision scientist. This has evolved with a strong focus on Machine Learning (ML) approaches to problem-solving, balancing normative and descriptive decision theories against behavioral economics, analysis and game theory.
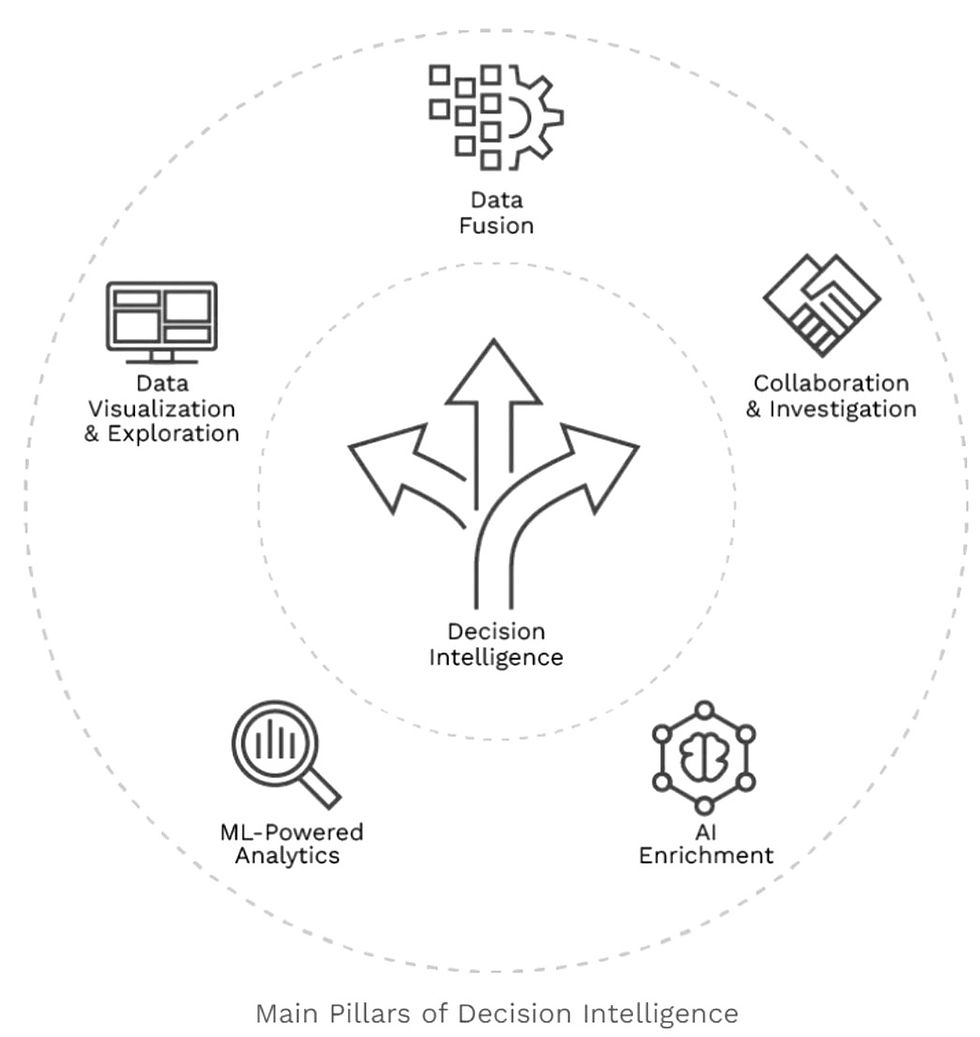
This has become increasingly interesting with the recent emergent capabilities of Large Language Models (LLMs) mimicking and performing logic. The performant logic of LLMs is heuristically derived from the training data of each model, which is a collection of "what this culture does in a given situation". Therefore it is reasonable to assume that LLMs would make reasonable decisions in most contexts, as this is how people make decisions daily, the very definition of heuristic.
Let's atomise DI concepts a little more, in the context of LLM augmentation.
Data Science and AI: Using machine learning and other AI techniques to analyze large volumes of data, identify patterns, and make predictions about future events. It will not be long before we have Data analytic agents that are capable of significantly augmenting capabilities, and there are developments in this field.
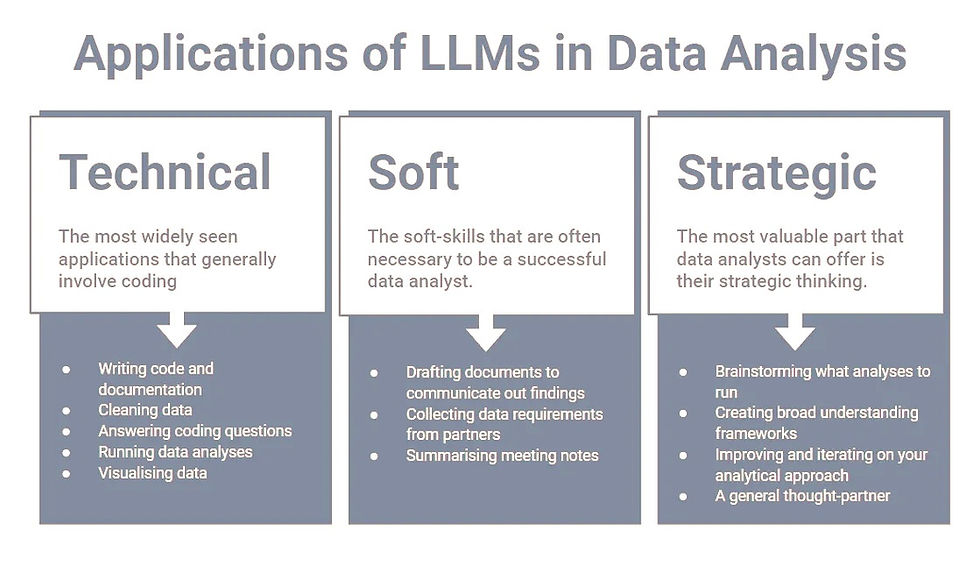
Behavioral and Social Science: Recognizing that decision-making is not just a rational process, but is also influenced by emotions, biases, social context, and other factors. LLMs being a reflection of the cultural data used in their training are exceptional tools in this domain, in a broader context. The specificities of individual stakeholders will require human understanding, as this is not automatable (yet).
Managerial Science: Understanding organizational structures and processes, managing resources efficiently, and coordinating teams and individuals. This may be the most challenging aspect for LLMs, as there is significant nuance and emotional understanding needed on the individual level. Fine-tuning an agent for managerial theory may not even be necessary as this is a part of the learning corpus. LLMs can take business case inputs and generate organizational processes, resource allocation plans, and team management suggestions based on analysis of data and past managerial science research.
Decision Engineering: This involves designing and building systems that can automate or assist with decision-making. These systems can range from simple rule-based systems to complex AI models and form a basis for current cognitive architectures in LLM reasoning. Chain of Thought (CoT), Tree of Thought (ToT) and Mixture of Experts (MoE - the cognitive architectural model behind GPT 4) are examples and applicable to engineered decision processes outside of LLM cognitive architecture, such as business decisions.
Learning Loops: Decision-making is a continuous process. Decisions lead to outcomes, which provide new data that can be used to refine future decisions. This cycle of decision-making, outcome measurement, and learning is sometimes called the "learning loop."
Visualization: Visual representations of data, models, and decisions to make them easier to understand and communicate.LLMs can take data and create a wide variety of visualizations like charts, graphs, and dashboards based on commands. Tools like Anthropic's Claude allow automatic visualization generation for instance, ChatGPT with plugins, and both Google Gemini, and OpenAIs GPT-4, have multi-modal capabilities (If the multi-model version of GPT 4 is ever released - I'm looking at you Sam).
Ethical Considerations: Issues related to privacy, fairness, transparency, and accountability. Researchers must provide LLMs with appropriate training data and guidelines so their outputs adhere to ethical principles. LLMs do not inherently consider ethics without explicit human direction. We should audit LLMs to ensure fairness, transparency and accountability.
As a general overview of this interesting field of cognitive science, there is exceptional room for growth in the application of AI for the best possible outcomes of multi-million, and multi-billion dollar decisions, derived systematically and scientifically. This has to be balanced with the knowledge that LLMs do not "think" or have the cognitive abilities to offer unique and new perspectives to problems, but very good approximations of what would generally be done in a given context. Until AI systems incorporate LLMs with a suite of abilities and tools, all of which are happening at a rapid rate, these systems cannot be trusted to make unguided or unmanaged decisions. They are extremely useful already though, and as the systems evolve and solve the key constraints of scrutability, confabulations, reliability and bias, will organisations be able to remain competitive without them?
Currently, I am working on various related models, tools and architectures for my master's degree final project and will publish a series based on the work being done.
コメント